A. Azizi, S.z. Shafaei, R. Rooki,
Volume 13, Issue 2 (6-2016)
Nowadays steel balls wear is a major problem in mineral processing industries and forms a significant part of the grinding cost. Different factors are effective on balls wear. It is needed to find models which are capable to estimate wear rate from these factors. In this paper a back propagation neural network (BPNN) and multiple linear regression (MLR) method have been used to predict wear rate of steel balls using some significant parameters including, pH, solid content, throughout of grinding circuit, speed of mill, charge weight of balls and grinding time. The comparison between the predicted wear rates and the measured data resulted in the correlation coefficients (R), 0.977 and 0.955 for training and test data using BPNN model. However, the R values were 0.936 and 0.969 for training and test data by MLR method. In addition, the average absolute percent relative error (AAPE) obtained 2.79 and 4.18 for train and test data in BPNN model, respectively. Finally, Analysis of the predictions shows that the BPNN and MLR methods could be used with good engineering accuracy to directly predict the wear rate of steel balls.
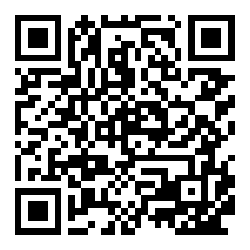